Quantifying the Quality of Haptic Interfaces
Shape-Changing Haptic Interfaces
Generating Clear Vibrotactile Cues with Magnets Embedded in a Soft Finger Sheath
Salient Full-Fingertip Haptic Feedback Enabled by Wearable Electrohydraulic Actuation
Cutaneous Electrohydraulic (CUTE) Wearable Devices for Pleasant Broad-Bandwidth Haptic Cues
Modeling Finger-Touchscreen Contact during Electrovibration
Perception of Ultrasonic Friction Pulses
Vibrotactile Playback for Teaching Sensorimotor Skills in Medical Procedures
CAPT Motor: A Two-Phase Ironless Motor Structure
4D Intraoperative Surgical Perception: Anatomical Shape Reconstruction from Multiple Viewpoints
Visual-Inertial Force Estimation in Robotic Surgery
Enhancing Robotic Surgical Training
AiroTouch: Naturalistic Vibrotactile Feedback for Large-Scale Telerobotic Assembly
Optimization-Based Whole-Arm Teleoperation for Natural Human-Robot Interaction
Finger-Surface Contact Mechanics in Diverse Moisture Conditions
Computational Modeling of Finger-Surface Contact
Perceptual Integration of Contact Force Components During Tactile Stimulation
Dynamic Models and Wearable Tactile Devices for the Fingertips
Novel Designs and Rendering Algorithms for Fingertip Haptic Devices
Dimensional Reduction from 3D to 1D for Realistic Vibration Rendering
Prendo: Analyzing Human Grasping Strategies for Visually Occluded Objects
Learning Upper-Limb Exercises from Demonstrations
Minimally Invasive Surgical Training with Multimodal Feedback and Automatic Skill Evaluation
Efficient Large-Area Tactile Sensing for Robot Skin
Haptic Feedback and Autonomous Reflexes for Upper-limb Prostheses
Gait Retraining
Modeling Hand Deformations During Contact
Intraoperative AR Assistance for Robot-Assisted Minimally Invasive Surgery
Immersive VR for Phantom Limb Pain
Visual and Haptic Perception of Real Surfaces
Haptipedia
Gait Propulsion Trainer
TouchTable: A Musical Interface with Haptic Feedback for DJs
Exercise Games with Baxter
Intuitive Social-Physical Robots for Exercise
How Should Robots Hug?
Hierarchical Structure for Learning from Demonstration
Fabrication of HuggieBot 2.0: A More Huggable Robot
Learning Haptic Adjectives from Tactile Data
Feeling With Your Eyes: Visual-Haptic Surface Interaction
S-BAN
General Tactile Sensor Model
Insight: a Haptic Sensor Powered by Vision and Machine Learning
Hierarchical Structure for Learning from Demonstration
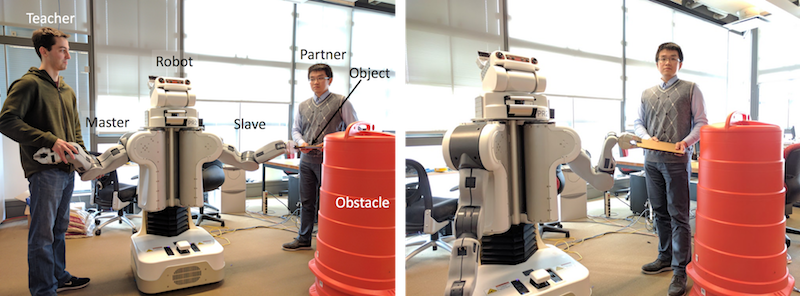
Many modern humanoid robots are designed to operate in human environments, like homes and hospitals. If designed well, such robots could help humans accomplish tasks and lower their physical and/or mental workload. As opposed to having an operator devise control policies and reprogram the robot for every new situation it encounters, learning from demonstration (LfD) provides a direct method for users to program robots.
We present a hierarchical structure of task-parameterized models for learning from demonstration: demonstrations from each situation are encoded in the same model, and models are combined to create a skill library. This structure has four advantages over the standard approach of encoding all demonstrations together: users can easily add or delete existing robot skills, robots can choose the most relevant skill to use for new tasks, robots can estimate the difficulty of these new test situations, and robot designers can apply domain knowledge to design better generalization behaviors.
We elucidate the proposed structure via application to a collaborative task where a robot arm moves an object with a human partner, as shown in the figure above. The demonstrations were collected for three situations using a novel kinesthetic teleoperation method [] and were encoded using task-parameterized Gaussian mixture models (TP-GMM). Naive participants then collaborated with the robot while it was controlled by a passive model with only gravity compensation, a single TP-GMM encoding all demonstrations, and the proposed structure. Our method achieved significantly better task performance and subjective ratings in most tested scenarios.
Members
Publications