Our research uses Computer Vision to learn digital humans that can perceive, learn, and act in virtual 3D worlds. This involves capturing the shape, appearance, and motion of real people as well as their interactions with each other and the 3D scene using monocular video. We leverage this to learn generative models of people and their behavior and evaluate these models by synthesizing realistic looking humans behaving in virtual worlds.
This work combines Computer Vision, Machine Learning, and Computer Graphics.
Director
Admin Team
Department Highlights
GraspXL: Generating Grasping Motions for Diverse Objects at Scale
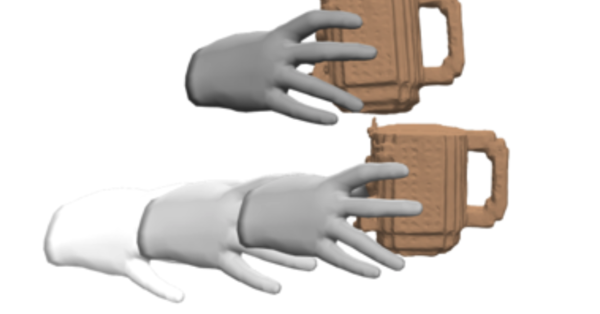
ContourCraft: Learning to Resolve Intersections in Neural Multi-Garment Simulations
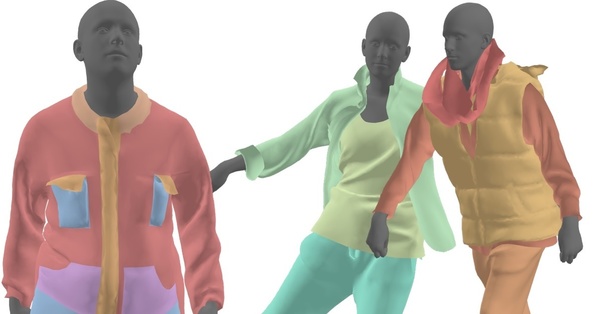
HOLD: Category-agnostic 3D Reconstruction of Interacting Hands and Objects from Video
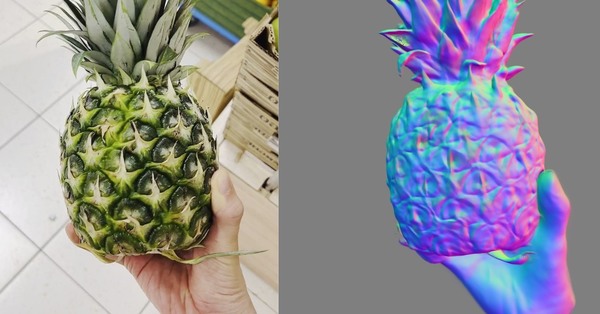
AMUSE: Emotional Speech-driven 3D Body Animation via Disentangled Latent Diffusion
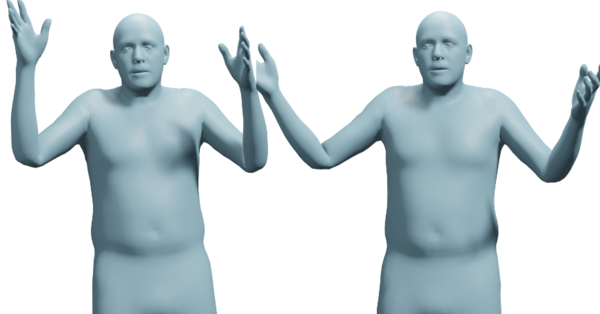