Human Pose, Shape and Action
3D Pose from Images
2D Pose from Images
Beyond Motion Capture
Action and Behavior
Body Perception
Body Applications
Pose and Motion Priors
Clothing Models (2011-2015)
Reflectance Filtering
Learning on Manifolds
Markerless Animal Motion Capture
Multi-Camera Capture
2D Pose from Optical Flow
Body Perception
Neural Prosthetics and Decoding
Part-based Body Models
Intrinsic Depth
Lie Bodies
Layers, Time and Segmentation
Understanding Action Recognition (JHMDB)
Intrinsic Video
Intrinsic Images
Action Recognition with Tracking
Neural Control of Grasping
Flowing Puppets
Faces
Deformable Structures
Model-based Anthropometry
Modeling 3D Human Breathing
Optical flow in the LGN
FlowCap
Smooth Loops from Unconstrained Video
PCA Flow
Efficient and Scalable Inference
Motion Blur in Layers
Facade Segmentation
Smooth Metric Learning
Robust PCA
3D Recognition
Object Detection
Faces
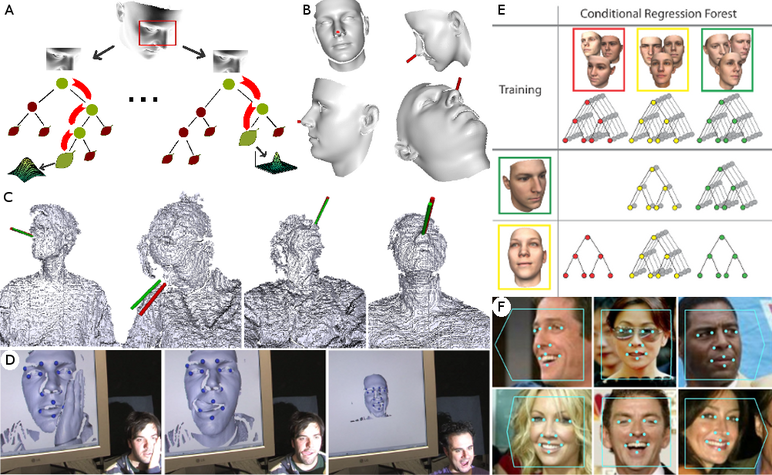
Due to its relevance for many applications like human computer interaction or face analysis, head pose estimation or facial feature point detection are very active areas in computer vision. Recent state-of-the-art methods have reported impressive results for these tasks where estimation accuracies of human annotators have been achieved. However, most of the available methods do not achieve real-time performance which is a requirement for many applications. We therefore address the problem of accurate head pose estimation or facial feature point detection in real-time from different modalities like images or depth data captured with a consumer depth camera.
To this end, we rely on a voting framework where patches extracted from the whole image can contribute to the estimation task. The intuition is that patches belonging to different parts of the image contain valuable information on global properties of the object which generated it, like pose. Since all patches can vote for the localization of a specific point of the object, it can be detected even when that particular point is occluded. To achieve real-time performance without the need of specific hardware like GPUs, the voting framework is implemented by random regression forests. Regression forests are a versatile tool for solving challenging computer vision tasks efficiently, being very fast at both train and test time, lend themselves to parallelization, and are inherently multi-class. Furthermore, they allow to find easily an optimal trade-off between accuracy and speed for a specific application. In this project, regression forests are used to learn a mapping from local image or depth patches to a probability over the parameter space, e.g., the 2D position of a facial feature point or the 3D orientation of the head.
Regression forests show their power when using large datasets, on which they can be trained efficiently. Because the accuracy of a regressor depends on the amount of annotated training data, the acquisition and labeling of a training set are key issues. Depending on the expected scenario, one can synthetically generate annotated depth images by rendering a face model undergoing large rotations, by recording real sequences using a consumer depth sensor and automatically annotating them using state-of-the-art tracking methods, or by crowd sourcing.
In the context of facial feature detection, regression forests tend to introduce a bias to the mean face since they learn the spatial relations between image patches and facial features from the complete training set and average the spatial distributions over all trees in the forest. In particular, patches that are not very close to a facial feature point favor the mean face, whereas patches close to a facial feature adapt better to local deformations but are more sensitive to occlusions. To find a good trade-off, the impact of the patches is steered based on the voting distance.
Another issue is the high intra-class variation of the data, which we address with the concept of conditional regression forests. In general, regression forests aim to learn the probability over the parameter space given a face image from the entire training set, where each tree is trained on a randomly sub-sampled training set to avoid over-fitting. The proposed conditional regression forests aim to learn several conditional probabilities over the parameter space instead. The motivation is that conditional probabilities are easier to learn since the trees do not have to deal with all facial variations in appearance and shape. Since some variations depend on global properties of the face like the head pose, the trees can be learned conditional to the global face properties. During training, the probability of the global properties are also learned to model the full probability over the parameter space. During testing, a set of pre-trained conditional regression trees is selected based on the estimated probability of the global properties. For instance, having trained regression trees conditional to various head poses, the probability of the head pose is estimated from the image and the corresponding trees are selected to predict the facial features. In this way, the trees that are selected for detecting facial features might vary from image to image. On a challenging database of faces captured ``in the wild'', the method achieves an accuracy comparable to the performance of human annotators in real-time.
Our current research is focused on extending the approach to profile faces and estimating occlusions of facial feature points as well.
Members
Publications