Human Pose, Shape and Action
3D Pose from Images
2D Pose from Images
Beyond Motion Capture
Action and Behavior
Body Perception
Body Applications
Pose and Motion Priors
Clothing Models (2011-2015)
Reflectance Filtering
Learning on Manifolds
Markerless Animal Motion Capture
Multi-Camera Capture
2D Pose from Optical Flow
Body Perception
Neural Prosthetics and Decoding
Part-based Body Models
Intrinsic Depth
Lie Bodies
Layers, Time and Segmentation
Understanding Action Recognition (JHMDB)
Intrinsic Video
Intrinsic Images
Action Recognition with Tracking
Neural Control of Grasping
Flowing Puppets
Faces
Deformable Structures
Model-based Anthropometry
Modeling 3D Human Breathing
Optical flow in the LGN
FlowCap
Smooth Loops from Unconstrained Video
PCA Flow
Efficient and Scalable Inference
Motion Blur in Layers
Facade Segmentation
Smooth Metric Learning
Robust PCA
3D Recognition
Object Detection
Neural Prosthetics and Decoding
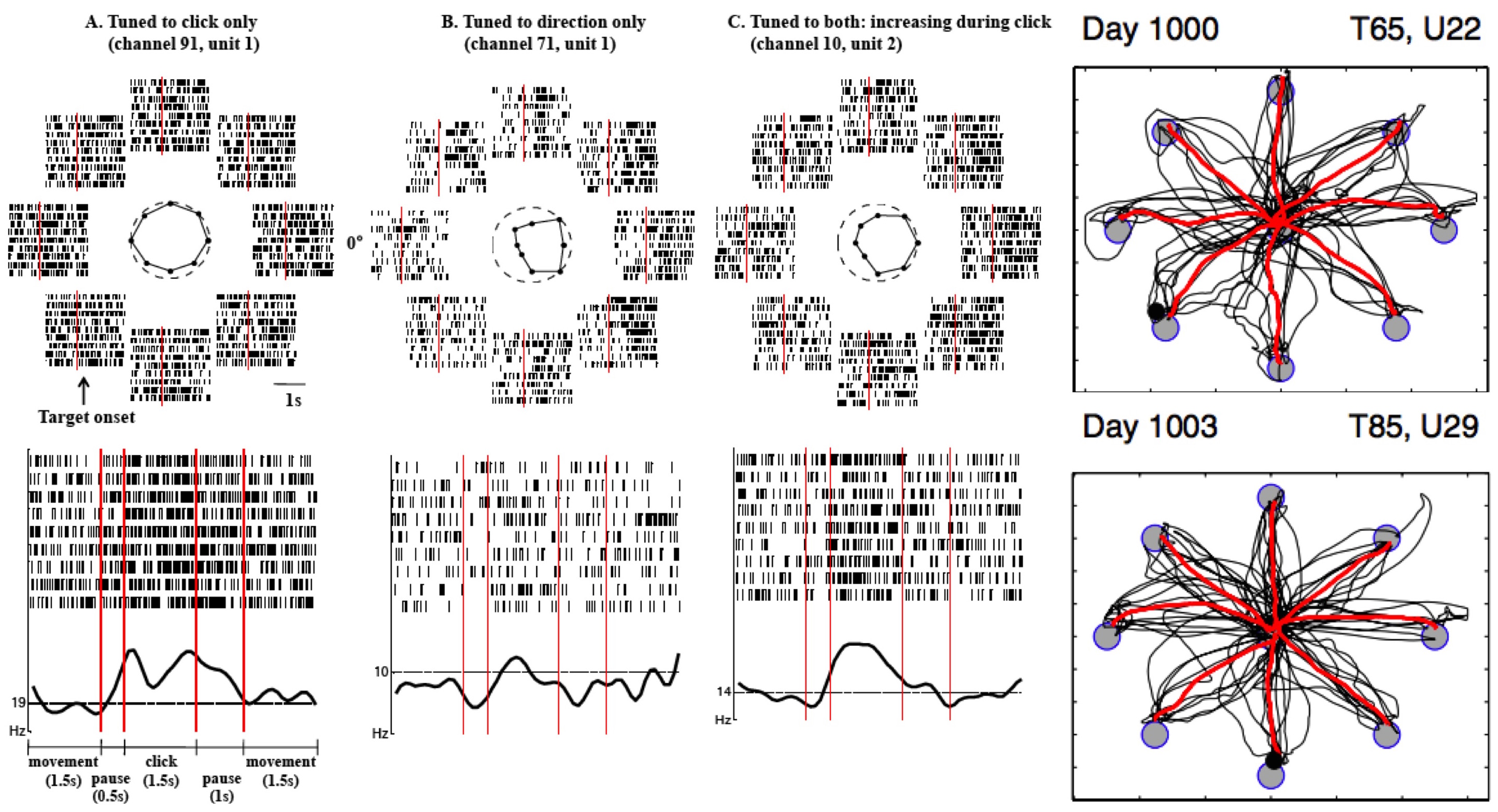
We use motion capture together with electrode arrays, implanted in the motor cortex of monkeys, to learn how motor cortical activity relates to movement and to create new algorithms to decode this activity. Translating these models to paralyzed humans allows us to restore or improve lost function in people with central nervous system injury by directly coupling brains with computers, allowing people to control a computer cursor with their thoughts.
We developed a point-and-click intracortical Brain Computer Interface (iBCI) that enables humans with tetraplegia to volitionally move a 2D computer cursor in any desired direction on a computer screen, hold it still, and click on an area of interest []. This direct brain-computer interface extracts both discrete (click) and continuous (cursor velocity) signals from a single small population of neurons in human motor cortex. Enabling this is a multi-state probabilistic decoding algorithm that simultaneously decodes neural spiking activity and outputs either a click signal or the velocity of the cursor. The algorithm combines a linear classifier, which determines whether the user is intending to click or move the cursor, with a Kalman filter that translates the neural population activity into cursor velocity. We present a paradigm for training the multi-state decoding algorithm using neural activity observed during imagined actions. We quantified point-and-click performance using various human-computer interaction measurements for pointing devices. We found that participants could control the cursor motion and click on specified targets, suggesting that signals from a small ensemble of motor cortical neurons (~40) can be used for natural point-and-click 2D cursor control of a personal computer. Furthermore in [
] we showed that such devices could be used to decode intended cursor movement over 1000 days after implantation.
Our ongoing work focuses on developing new non-linear decoding algorithms [].
Members
Publications