Human Pose, Shape and Action
3D Pose from Images
2D Pose from Images
Beyond Motion Capture
Action and Behavior
Body Perception
Body Applications
Pose and Motion Priors
Clothing Models (2011-2015)
Reflectance Filtering
Learning on Manifolds
Markerless Animal Motion Capture
Multi-Camera Capture
2D Pose from Optical Flow
Body Perception
Neural Prosthetics and Decoding
Part-based Body Models
Intrinsic Depth
Lie Bodies
Layers, Time and Segmentation
Understanding Action Recognition (JHMDB)
Intrinsic Video
Intrinsic Images
Action Recognition with Tracking
Neural Control of Grasping
Flowing Puppets
Faces
Deformable Structures
Model-based Anthropometry
Modeling 3D Human Breathing
Optical flow in the LGN
FlowCap
Smooth Loops from Unconstrained Video
PCA Flow
Efficient and Scalable Inference
Motion Blur in Layers
Facade Segmentation
Smooth Metric Learning
Robust PCA
3D Recognition
Object Detection
DRAPE: DRessing Any PErson
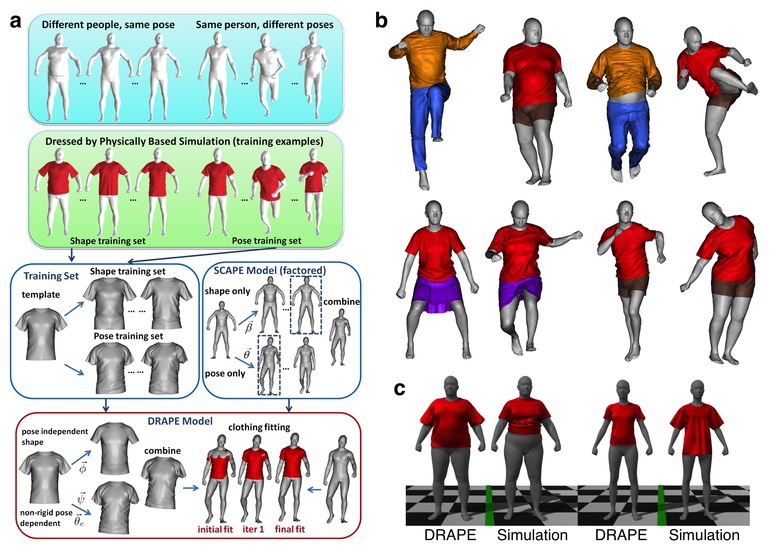
Clothed virtual characters in varied sizes and shapes are necessary for film, gaming, and on-line fashion applications. Dressing such characters is a significant bottleneck, requiring manual effort to design clothing, position it on the body, and simulate its physical deformation.
To address this, we have developed a complete system for animating realistic clothing on synthetic bodies of any shape and pose without manual intervention. DRAPE is learned from standard 2D clothing designs simulated on 3D avatars with varying shape and pose. Once learned, DRAPE adapts to different body shapes and poses without the redesign of clothing patterns; this effectively creates infinitely-sized clothing. A key contribution is that the method is automatic. In particular, animators do not need to place the cloth pieces in appropriate positions to dress an avatar.
The key component of the method is a model of clothing called DRAPE (DRessing Any PErson) that is learned from a physics-based simulation of clothing on bodies of different shapes and poses. The DRAPE model has the desirable property of “factoring” clothing deformations due to body shape from those due to pose variation. This factorization provides an approximation to the physical clothing deformation and greatly simplifies clothing synthesis.
We start with a parameterized model of the 3D human body represented using SCAPE. Given the shape and pose parameters describing the body, the DRAPE algorithm dresses the body with a garment that is customized to fit and possesses realistic wrinkles. There a three main steps:
1. Training: The shape training set consists of SCAPE bodies with different shapes in the same pose. The pose training set contains a single body shape moving through sequences of poses. For each training body shape, we manually choose a size for each garment and dress the body using physics-based simulation (Figure a, row 2).
2. Learned clothing deformation model: For each garment, we learn a factored clothing model that represents: i) rigid rotation of the cloth pieces, e.g. the rotation of a sleeve w. r. t. the torso; ii) pose-independent clothing shape variations, that are linearly predicted from the underlying body shape (learned from the shape training set); and iii) pose-dependent non-rigid deformations, that are linearly predicted from a short history of body poses and clothing shapes (learned from the pose training set).
3. Virtual fitting: Using the pose-independent model, we map body shape parameters to clothing shape parameters to obtain a custom shaped garment for a given body. Clothing parts are associated with body parts and the pose of the body is applied to the garment parts by rigid rotation. The learned model of pose-dependent wrinkles is then applied. The custom garment is automatically aligned with the body and interpenetration between the garment and the body is removed by efficiently solving a system of linear equations.
DRAPE can be used to dress static bodies or animated sequences with a learned model of the cloth dynamics. Since the method is fully automated, it is appropriate for dressing large numbers of virtual characters of varying shape. The method is significantly more efficient than physical simulation. This makes it appropriate for web applications such as virtual clothing try-on.
Video
Members
Publications