Research Overview
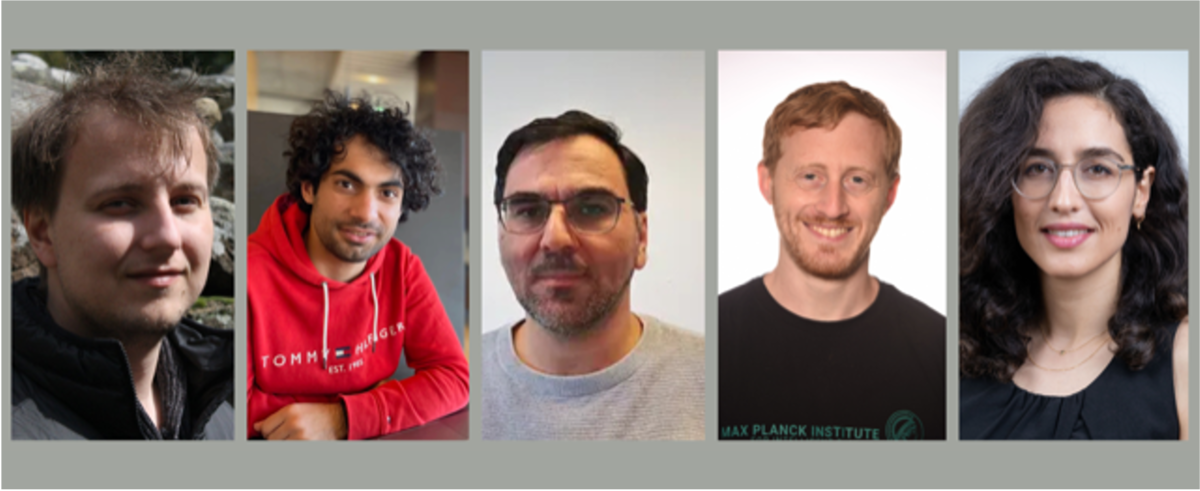
Machine learning is transforming decision-making in healthcare, finance, and AI-assisted recommendations. However, its integration with human behavior presents challenges in efficiency, adaptability, interpretability, and fairness. We examine how ML models influence human decisions and vice versa, showing that collaborative systems can settle into suboptimal equilibria. Additionally, we design novel frameworks to enhance the performance of these systems by addressing fairness, robustness, and counterfactual explanations. These findings contribute to making ML systems more ethical, adaptive, and better aligned with real-world needs.
Machine learning is transforming decision-making in many tasks we encounter in our daily lives. While these systems offer efficiency and automation, they also present challenges related to adaptability, fairness, interpretability, and human-AI collaboration. Our research explores how machine learning interacts with human decision-makers—both statically and over time—to ensure accurate, ethical, and interpretable outcomes.
One challenge arises when machine learning models influence human decisions in ways that lead to suboptimal outcomes over time. The interaction between humans and ML systems can create unintended patterns or even degrade accuracy. To address this, we aim to design human-ML collaboration systems that enhance each other’s strengths. Beyond accuracy and performance, ML systems must also make ethical decisions that are resistant to manipulation. Achieving this requires integrating fairness, adversarial robustness, and causal reasoning into model design. Additionally, decision-making systems should consider broader societal implications, ensuring recommendations are not only personalized but also equitable and transparent.
Another critical issue is interpretability. AI-generated outputs, such as those from large language models, do not always follow human-like reasoning, raising fundamental questions about how these models should be understood and evaluated. As discussions about general artificial intelligence become more common, researchers have increasingly applied human-centered evaluation methods to large language models—often without proper adaptation. Blindly transferring human-centered measures to AI can lead to misleading conclusions, making it essential to develop better frameworks for assessing AI behavior.
By addressing these challenges, our research contributes to building machine learning systems that are more reliable, fair, and better collaborators for humans. Ensuring that AI effectively supports human decision-makers is essential for the responsible development and deployment of these technologies.