Empirical Inference
Members
Publications
Visualization of the machine learning approach to uncover critical features. Consider the problem of predicting for an image patch whether it is likely to be a saccade target or not. Panel (a) depicts image patches that have (yellow) or have not (red) been saccade targets. The yellow-to-red gradient represent the response of a SVM with a Gaussian kernel trained on the patches. Panel (b): Gradient descent on the function that the SVM implements, extrema are indicated. Panel (c): Using the extrema, a network with four kernels centered on them approximates the SVM. (Adapted from [
].)
]. We wrote a software package to perform Bayesian inference for the psychometric function for non-stationary data. In addition, we introduced the use of spatial point processes to characterize eye-movement fixation patterns [
]. Finally, we continue to improve machine learning methods to uncover the critical features observers use in complex perceptual tasks [
]) (c.f. [
]).
].


].
]
Psychophysics and Computational Models of Behaviour
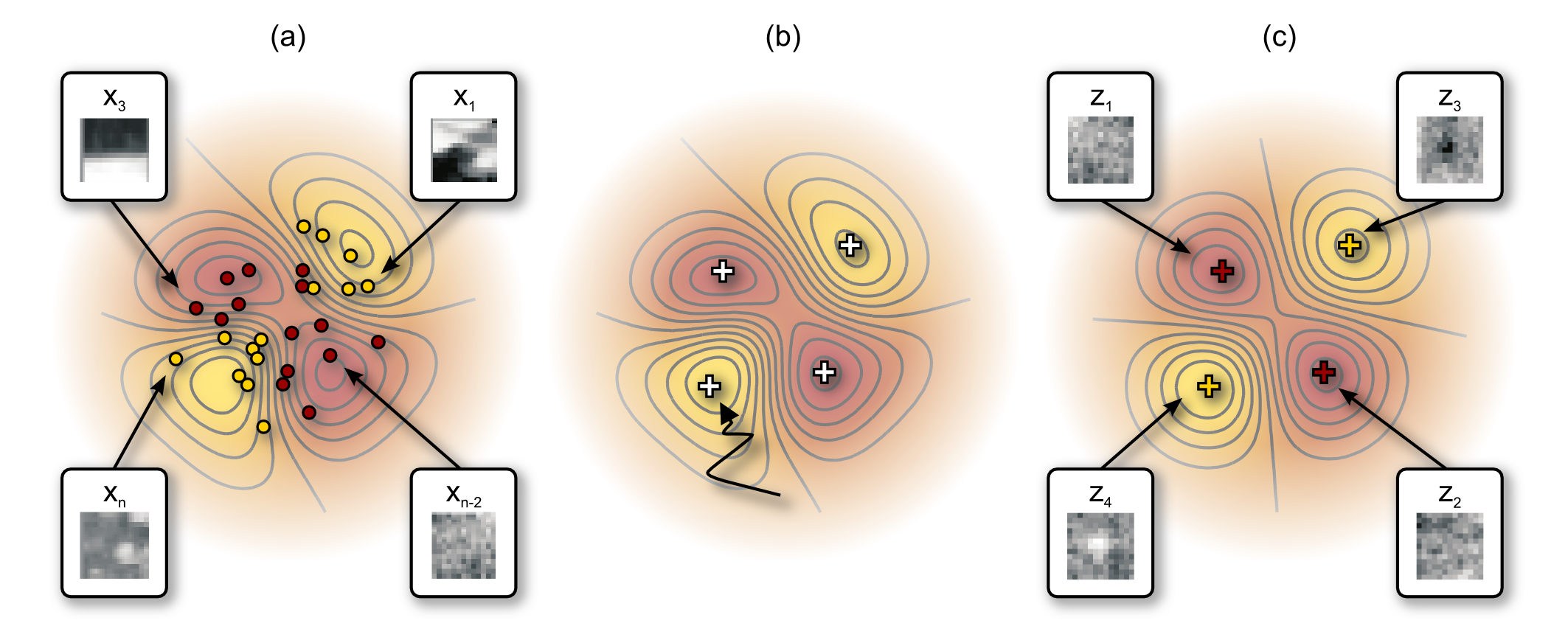
This project investigates human perception, combining psychophysical experiments and computational modeling. Currently we have four main research foci:
First, we develop methods to gain more information from psychophysical data. We characterized serial dependencies in behavioral responses, and introduced methods to correct for them [
Our second focus is the development of an image-based model of spatial vision. We integrated the large psychophysical literature on simple detection and discrimination experiments and proposed a model based on maximum-likelihood decoding of a population of model neurons predicting several data sets simultaneously, using a single set of parameters [
Third, the project seeks to understand the fundamental questions of characterizing the computational principles underlying lightness perception, treating lightness perception as one example of how to rigorously study the visual mechanisms translating ambiguous retinal input into perceptually and psychologically relevant categories [
Finally, we are moving towards understanding perceptual causality. Recently there has been considerable progress in understanding causal inference by viewing it as a machine learning problem. This is relevant to perception since animals cannot operate based on the assumption of independent and identically distributed (iid) data but need to employ suitable inference methods that work under changing distributions in order to produce robust perception. We study causal models of perception in a simple binary classification setting where the perceiver needs to distinguish cause and effect, or forward and backward, a task we recently studied in a computer vision context [
Members
Publications
Empirical Inference
Article
Noise masking of White’s illusion exposes the weakness of current spatial filtering models of lightness perception
Betz, T., Shapley, R. M., Wichmann, F. A., Maertens, M.
Journal of Vision, 15(14):1-17, October 2015 (Published)
DOI
BibTeX
Empirical Inference
Article
Testing the role of luminance edges in White’s illusion with contour adaptation
Betz, T., Shapley, R. M., Wichmann, F. A., Maertens, M.
Journal of Vision, 15(11):1-16, August 2015 (Published)
DOI
BibTeX
Empirical Inference
Article
Spatial statistics and attentional dynamics in scene viewing
Engbert, R., Trukenbrod, H., Barthelmé, S., Wichmann, F.
Journal of Vision, 15(1):1-17, 2015 (Published)
Web
PDF
DOI
URL
BibTeX
Empirical Inference
Article
Quantifying the effect of intertrial dependence on perceptual decisions
Fründ, I., Wichmann, F., Macke, J.
Journal of Vision, 14(7):1-16, 2014 (Published)
Web
PDF
DOI
URL
BibTeX
Empirical Inference
Article
How Sensitive Is the Human Visual System to the Local Statistics of Natural Images?
Gerhard, H., Wichmann, F., Bethge, M.
PLoS Computational Biology, 9(1):e1002873, January 2013 ()
DOI
BibTeX
Empirical Inference
Article
A neural population model for visual pattern detection
Goris, R., Putzeys, T., Wagemans, J., Wichmann, F.
Psychological Review, 120(3):472–496, 2013 ()
DOI
BibTeX
Empirical Inference
Article
Identification of stimulus cues in narrow-band tone-in-noise detection using sparse observer models
Schönfelder, V., Wichmann, F.
Journal of the Acoustical Society of America, 134(1):447-463, 2013 ()
DOI
BibTeX
Empirical Inference
Article
Modeling fixation locations using spatial point processes
Barthelmé, S., Trukenbrod, H., Engbert, R., Wichmann, F.
Journal of Vision, 13(12):1-34, 2013 ()
Web
DOI
BibTeX
Empirical Inference
Article
When luminance increment thresholds depend on apparent lightness
Maertens, M., Wichmann, F.
Journal of Vision, 13(6):1-11, 2013 ()
DOI
BibTeX
Empirical Inference
Article
Sparse regularized regression identifies behaviorally-relevant stimulus features from psychophysical data
Schönfelder, V., Wichmann, F.
Journal of the Acoustical Society of America, 131(5):3953-3969, May 2012 ()
Web
DOI
BibTeX
Empirical Inference
Article
A New Perceptual Bias Reveals Suboptimal Population Decoding of Sensory Responses
Putzeys, T., Bethge, M., Wichmann, F., Wagemans, J., Goris, R.
PLoS Computational Biology, 8(4):1-13, April 2012 ()
Web
DOI
BibTeX
Empirical Inference
Article
Estimating predictive stimulus features from psychophysical data: The decision image technique applied to human faces
Macke, J., Wichmann, F.
Journal of Vision, 10(5:22):1-24, May 2010 ()
Web
DOI
BibTeX
Empirical Inference
Article
Does Cognitive Science Need Kernels?
Jäkel, F., Schölkopf, B., Wichmann, F.
Trends in Cognitive Sciences, 13(9):381-388, September 2009 ()
PDF
Web
DOI
BibTeX
Empirical Inference
Article
Center-surround patterns emerge as optimal predictors for human saccade targets
Kienzle, W., Franz, M., Schölkopf, B., Wichmann, F.
Journal of Vision, 9(5:7):1-15, May 2009 ()
PDF
DOI
BibTeX