Optimization and Large Scale Learning
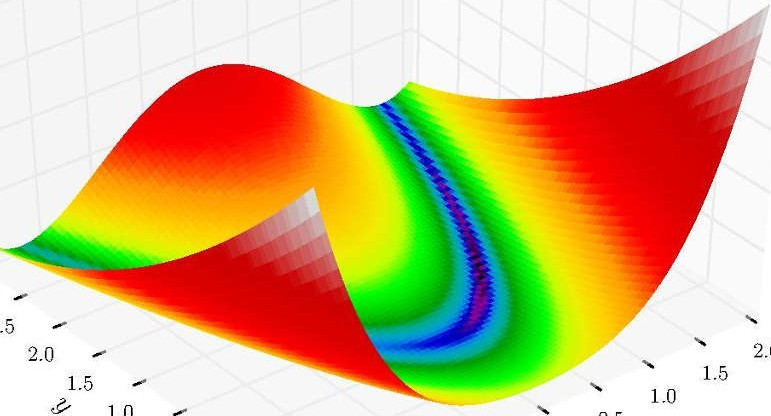
Optimization lies at the heart of most machine learning algorithms. The key aspects of the applications in which these algorithms are applied include: high-dimensional, noisy, and uncertain data; huge volumes of batch or streaming data; intractable models, low accuracy, and reliance on distributed computation or stochastic approximations. The success of most machine learning algorithms depends on how the optimization techniques can adapt and exploit these facets. Our interests are broadly divided into two categories, convex and non-convex methods.
Convex optimization In the realm of methods for convex optimization, we have addressed research challenges under various different problem settings. For large-scale problems, where scalability is an important aspect, a summary overview of large-scale aspects of convex optimization appears in our work []. A theoretically optimal large-scale convex method for problems with linear constraints is presented in [
] which develops a new stochastic alternating direction method of multipliers (ADMM)
method that combines Nesterov's accelerated gradient methods with ADMM.
For learning classifiers in extremely large output spaces, we have proposed a parallelizable mixed-norm regularization approach leading to convex but non-smooth optimization in our recent work []. We show that the resulting models can be orders of magnitude smaller than most state-of-the-art methods and also lead to better generalization performance.
A contribution that lies at the interface of combinatorial and convex optimization is presented in []. General purpose convex methods often rely on key subroutines such as projection and proximity operators. Continuing our effort from the previous SAB assessment towards developing a library of highly tuned subroutines, e.g., for total variation, we extend to multivariate total variation in [
]. A flourishing sub-field of convex optimization is ``Robust optimization'' which seeks to optimize models under parameters/data uncertainty. It intersects with the usual min-max theory in statistics, and can be used to offer a different view of regularization in machine learning. In [
], we introduced the notion of robust optimization for matrix completion/recovery problems. The actual application was to recover correlation matrices under a simple bounded uncertainty model.
Non-convex Optimization In the domain of non-convex optimization for large-scale problems, our work [] presents a simplified analysis of what, to our knowledge, is the first non-convex, non-smooth incremental proximal method. This work started in 2011; interestingly, in recent years, the interest in incremental methods has sky-rocketed, though the analysis is limited only to the convex case. Finally, we mention a new direction in nonconvex optimization offered by our recent work [
], which introduces "Geometric optimization" on the manifold of positive definite matrices. The underlying idea is to develop a theory of convexity along geodesics on the Positive Semi-Definite manifold. This work also identifies some basic calculus rules for detection and construction of geodesically convex functions on the Positive Definite manifold, and as an application presents new algorithms for solving maximum likelihood estimation for elliptically contoured distributions, which despite non-convexity remain tractable thanks to geodesic convexity.
Members
Publications