Robust Affordable 3D Haptic Sensation via Learning Deformation Patterns
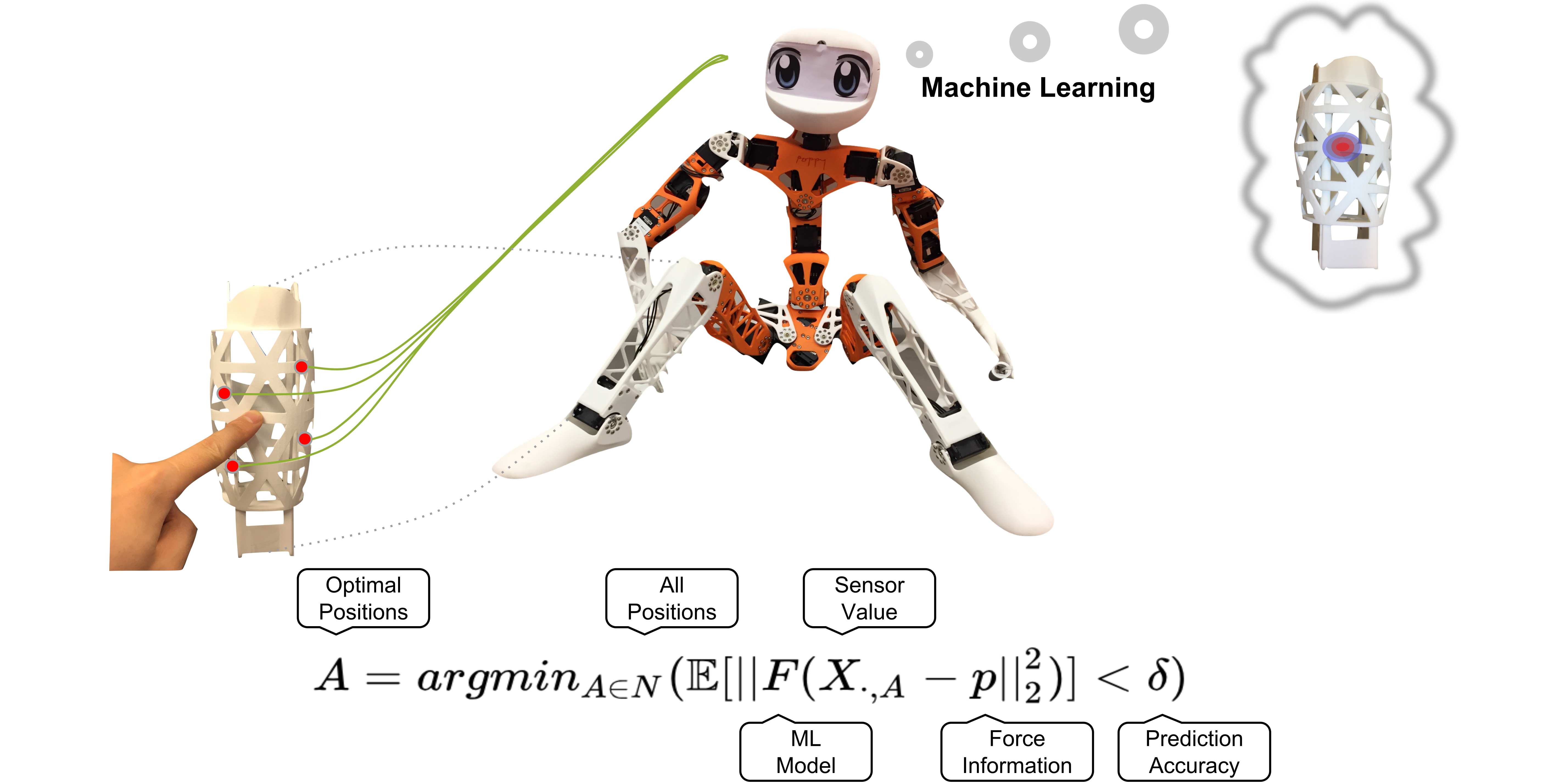
Haptic sensation is an important modality for interacting with the real world. This paper proposes a general framework of inferring haptic forces on the surface of a 3D structure from internal deformations using a small number of physical sensors instead of employing dense sensor arrays. Using machine learning techniques, we optimize the sensor number and their placement and are able to obtain high-precision force inference for a robotic limb using as few as 9 sensors. For the optimal and sparse placement of the measurement units (strain gauges), we employ data-driven methods based on data obtained by finite element simulation. We compare data-driven approaches with model-based methods relying on geometric distance and information criteria such as Entropy and Mutual Information. We validate our approach on a modified limb of the “Poppy” robot [1] and obtain 8 mm localization precision.
Author(s): | Huanbo Sun and Georg Martius |
Book Title: | Proceedings International Conference on Humanoid Robots |
Pages: | 846-853 |
Year: | 2018 |
Publisher: | IEEE |
Project(s): | |
Bibtex Type: | Conference Paper (conference) |
Address: | New York, NY, USA |
DOI: | 10.1109/HUMANOIDS.2018.8625064 |
Event Name: | 2018 IEEE-RAS International Conference on Humanoid Robots |
Event Place: | Peking, China |
Electronic Archiving: | grant_archive |
Note: | Oral Presentation |
BibTex
@conference{SunMartius2018:SingleTouchSensation, title = {Robust Affordable 3D Haptic Sensation via Learning Deformation Patterns}, booktitle = {Proceedings International Conference on Humanoid Robots}, abstract = {Haptic sensation is an important modality for interacting with the real world. This paper proposes a general framework of inferring haptic forces on the surface of a 3D structure from internal deformations using a small number of physical sensors instead of employing dense sensor arrays. Using machine learning techniques, we optimize the sensor number and their placement and are able to obtain high-precision force inference for a robotic limb using as few as 9 sensors. For the optimal and sparse placement of the measurement units (strain gauges), we employ data-driven methods based on data obtained by finite element simulation. We compare data-driven approaches with model-based methods relying on geometric distance and information criteria such as Entropy and Mutual Information. We validate our approach on a modified limb of the “Poppy” robot [1] and obtain 8 mm localization precision.}, pages = {846-853}, publisher = {IEEE}, address = {New York, NY, USA}, year = {2018}, note = {Oral Presentation}, slug = {sunmartius2018-singletouchsensation}, author = {Sun, Huanbo and Martius, Georg} }