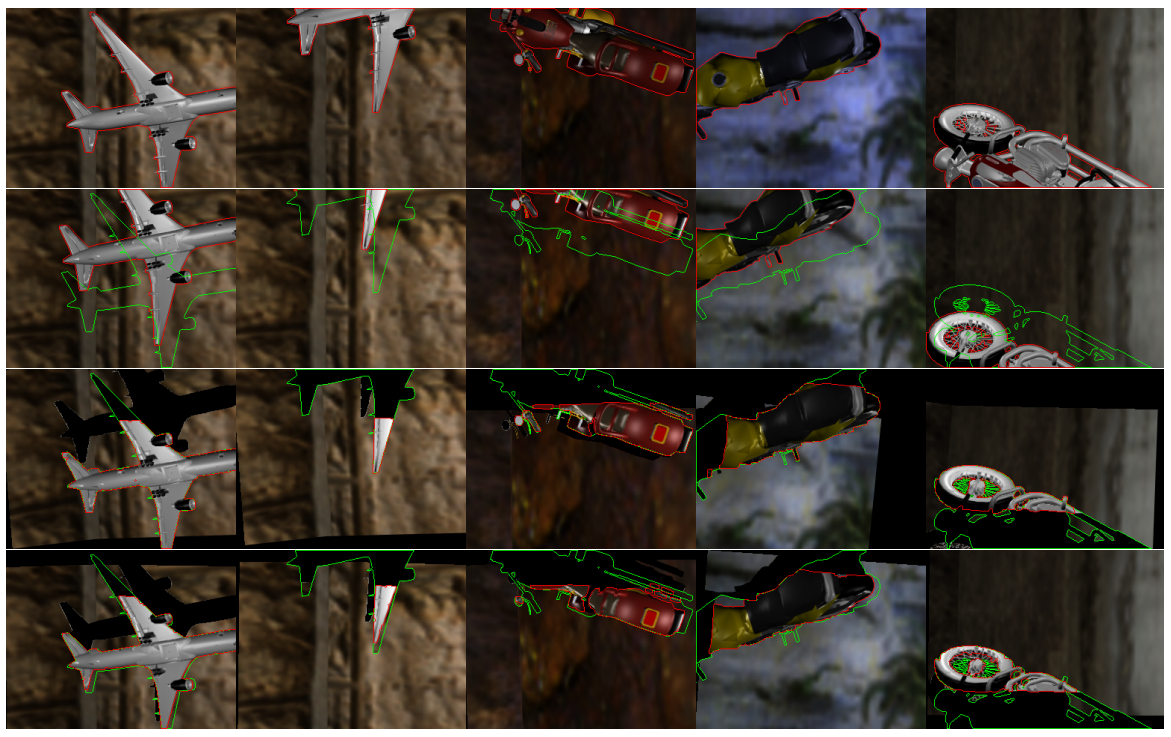
In this paper, we provide a modern synthesis of the classic inverse compositional algorithm for dense image alignment. We first discuss the assumptions made by this well-established technique, and subsequently propose to relax these assumptions by incorporating data-driven priors into this model. More specifically, we unroll a robust version of the inverse compositional algorithm and replace multiple components of this algorithm using more expressive models whose parameters we train in an end-to-end fashion from data. Our experiments on several challenging 3D rigid motion estimation tasks demonstrate the advantages of combining optimization with learning-based techniques, outperforming the classic inverse compositional algorithm as well as data-driven image-to-pose regression approaches.
Author(s): | Zhaoyang Lv and Frank Dellaert and James M. Rehg and Andreas Geiger |
Book Title: | Proceedings IEEE Conf. on Computer Vision and Pattern Recognition (CVPR) |
Year: | 2019 |
Month: | June |
Bibtex Type: | Conference Paper (inproceedings) |
Event Name: | IEEE International Conference on Computer Vision and Pattern Recognition (CVPR) 2019 |
Event Place: | Long Beach, USA |
Electronic Archiving: | grant_archive |
Links: |
BibTex
@inproceedings{Lv2019CVPR, title = {Taking a Deeper Look at the Inverse Compositional Algorithm}, booktitle = {Proceedings IEEE Conf. on Computer Vision and Pattern Recognition (CVPR)}, abstract = {In this paper, we provide a modern synthesis of the classic inverse compositional algorithm for dense image alignment. We first discuss the assumptions made by this well-established technique, and subsequently propose to relax these assumptions by incorporating data-driven priors into this model. More specifically, we unroll a robust version of the inverse compositional algorithm and replace multiple components of this algorithm using more expressive models whose parameters we train in an end-to-end fashion from data. Our experiments on several challenging 3D rigid motion estimation tasks demonstrate the advantages of combining optimization with learning-based techniques, outperforming the classic inverse compositional algorithm as well as data-driven image-to-pose regression approaches.}, month = jun, year = {2019}, slug = {lv2019cvpr}, author = {Lv, Zhaoyang and Dellaert, Frank and Rehg, James M. and Geiger, Andreas}, month_numeric = {6} }