Benchmarking Offline Reinforcement Learning on Real-Robot Hardware
Website arXiv Code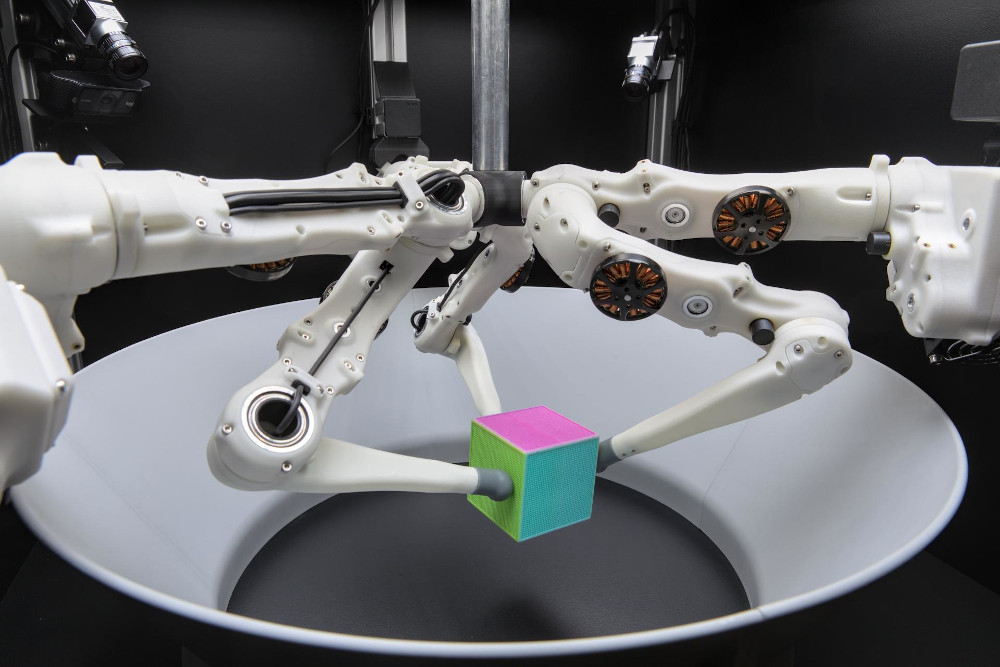
Learning policies from previously recorded data is a promising direction for real-world robotics tasks, as online learning is often infeasible. Dexterous manipulation in particular remains an open problem in its general form. The combination of offline reinforcement learning with large diverse datasets, however, has the potential to lead to a breakthrough in this challenging domain analogously to the rapid progress made in supervised learning in recent years. To coordinate the efforts of the research community toward tackling this problem, we propose a benchmark including: i) a large collection of data for offline learning from a dexterous manipulation platform on two tasks, obtained with capable RL agents trained in simulation; ii) the option to execute learned policies on a real-world robotic system and a simulation for efficient debugging. We evaluate prominent open-sourced offline reinforcement learning algorithms on the datasets and provide a reproducible experimental setup for offline reinforcement learning on real systems.
Author(s): | Nico Gürtler and Sebastian Blaes and Pavel Kolev and Felix Widmaier and Manuel Wüthrich and Stefan Bauer and Bernhard Schölkopf and Georg Martius |
Links: | |
Book Title: | Proceedings of the Eleventh International Conference on Learning Representations |
Year: | 2023 |
Month: | May |
Day: | 1-5 |
Bibtex Type: | Conference Paper (inproceedings) |
Event Name: | The Eleventh International Conference on Learning Representations (ICLR) |
Event Place: | Rwanda, Africa |
State: | Published |
URL: | https://openreview.net/forum?id=3k5CUGDLNdd |
Electronic Archiving: | grant_archive |
Talk Type: | Oral (notable-top-25%) |
BibTex
@inproceedings{benchmarkingofflinerl, title = {Benchmarking Offline Reinforcement Learning on Real-Robot Hardware}, booktitle = {Proceedings of the Eleventh International Conference on Learning Representations}, abstract = {Learning policies from previously recorded data is a promising direction for real-world robotics tasks, as online learning is often infeasible. Dexterous manipulation in particular remains an open problem in its general form. The combination of offline reinforcement learning with large diverse datasets, however, has the potential to lead to a breakthrough in this challenging domain analogously to the rapid progress made in supervised learning in recent years. To coordinate the efforts of the research community toward tackling this problem, we propose a benchmark including: i) a large collection of data for offline learning from a dexterous manipulation platform on two tasks, obtained with capable RL agents trained in simulation; ii) the option to execute learned policies on a real-world robotic system and a simulation for efficient debugging. We evaluate prominent open-sourced offline reinforcement learning algorithms on the datasets and provide a reproducible experimental setup for offline reinforcement learning on real systems.}, month = may, year = {2023}, slug = {benchmarkingofflinerl}, author = {G{\"u}rtler, Nico and Blaes, Sebastian and Kolev, Pavel and Widmaier, Felix and W{\"u}thrich, Manuel and Bauer, Stefan and Sch{\"o}lkopf, Bernhard and Martius, Georg}, url = {https://openreview.net/forum?id=3k5CUGDLNdd}, month_numeric = {5} }